Democratic Input and Artificial Intelligence
Governing with AI: Living Literature Review Post 2
Overview
In this Living Literature Review, I will guide you on an examination of how digital tools and advanced AI systems are already altering how democratic input is both generated and delivered to governments. We will begin with a brief history of voting methods in the US as an example of how technological evolution has historically shaped this particular type of democratic input. From here we explore two recent academic literature reviews from late 2024 on the topics of digital tools for citizen participation and the use of LLMs in politics and democracy. These literature reviews provide rich material from which we can explore more deeply on these topics. Then we explore, in detail, two specific empirical studies from 2024 that apply LLMs to the challenges of low voter turnout and low information on collective preferences. Both of these studies point to promising specific directions for LLMs to improve democratic input, but also highlight that LLMs are not ready to be “digital twins” or strong predict individual policy preferences. Finally, as something of a robustness check, we explore the recently released 2024 Federal AI Use Case Inventory to see how US Federal Agencies are developing and deploying LLMs to improve democratic input, with a specific focus on simulating collective preferences and behaviors as they relate to various policy domains.
Introduction
One of the key governing strengths of a democracy is democratic input. Democratic input provides the feedback needed for the governing system to respond, adapt, and evolve. Democratic input also sets the general direction of the goals of a functioning democracy. Democratic input captures the sense of democracy that Lincoln pointed at in his Gettysburg Address: “Government of the people, by the people, for the people”. However, as with bureaucracies1, democratic input has evolved alongside technological and cultural evolution.
One well documented example of this in the US context (the one with which I am most familiar) is voting. Voting is the mechanism that is most often associated with democratic input. In the US, voting methods are diverse, as they are run by individual states, but nonetheless one can see a broad trend of technological evolution from the late 1800’s until now.2 The broad strokes are that voting went from using your voice in a public setting, to submitting party-backed pre-filled ballots, to standardized paper ballots, to machines with a lever that you used to mark paper ballots, to touchscreen digital electronic screens with paper trails, to, finally, secure apps and web-portals. You can see representative pictures of several of these types here in Douglas Jones (2003) “A Brief Illustrated History of Voting.” I’ve included a few these pictures below:
![[photo of a general election ballot] [photo of a general election ballot]](https://substackcdn.com/image/fetch/w_1456,c_limit,f_auto,q_auto:good,fl_lossy/https%3A%2F%2Fsubstack-post-media.s3.amazonaws.com%2Fpublic%2Fimages%2F5b636a94-8dc5-4c78-a85c-4b6f2353b2e6_180x388.gif)
![[photo of a partisan general election ballot] [photo of a partisan general election ballot]](https://substackcdn.com/image/fetch/w_1456,c_limit,f_auto,q_auto:good,fl_progressive:steep/https%3A%2F%2Fsubstack-post-media.s3.amazonaws.com%2Fpublic%2Fimages%2Fab076871-65b7-4fb0-a4eb-3f54fb753c92_230x364.gif)
![[photo] [photo]](https://substackcdn.com/image/fetch/w_1456,c_limit,f_auto,q_auto:good,fl_progressive:steep/https%3A%2F%2Fsubstack-post-media.s3.amazonaws.com%2Fpublic%2Fimages%2F5aa1de3e-51e0-4b86-b0bc-3314c1e96934_1429x960.jpeg)
![[photo] [photo]](https://substackcdn.com/image/fetch/w_1456,c_limit,f_auto,q_auto:good,fl_progressive:steep/https%3A%2F%2Fsubstack-post-media.s3.amazonaws.com%2Fpublic%2Fimages%2Fc2798f29-8579-479b-952a-6b07c4cf95a6_1025x1472.jpeg)
While this evolution of voting is instructive, it is only one piece of the evolution of democratic participation towards more use of newer information and communication technologies (ICTs) and “electronic” participation (e-participation) tools.
In the following section, we begin with an overview of the standard “tools” of the “current paradigm” of democratic input from Shin et al’s (2024) “A systematic analysis of digital tools for citizen participation.” With the stage set for an understanding of how digital tools have already reshaped democratic input and citizen participation, we will then review the current evidence for the use of advanced AI, typically in the form of Large Language Models (LLMs) for democratic input. For this, we begin with an exploration of Aoki’s (2024) “Large Language Models in Politics and Democracy: A Comprehensive Survey.” This survey provides an overview from the academic literature of the ways in which LLMs are already being used as forms of democratic input. Then we will explore two specific studies that look at using LLMs for soliciting individual policy preferences, individual votes, and simulating collective preferences as captured by elections. Finally, we briefly explore the recently released 2024 US Federal AI Use Case Inventory for further examples of LLM use by US Federal Agencies to improve democratic input.
A Systematic Analysis of Digital Tools for Citizen Participation
The first of the two academic literature reviews from 2024 that we will explore is “A Systematic Analysis of Digital Tools for Citizen Participation.” Shin et. al. both give us a framework for thinking about digital participatory tools and analyze 116 tools.
This article aligns with the approach by systematically analysing digital tools in the context of e-participation. Digital participatory tools are defined as digital applications or systems specifically designed to enhance e-participation. Consequently, general tools such as Facebook and Zoom are excluded from the scope of this article. Instead of focusing on isolated instances, this article identifies prominent trends by analysing a comprehensive dataset of 116 digital participatory tools from three public repositories.
In the article, the authors raise three guiding research questions:
What are the main functions that digital tools provide for e-participation?
What are the prominent clusters of digital tools?
Do digital tools potentially enhance inclusiveness, deliberation, and empowerment?
The study goes on to identify specific “genes” across four dimensions that can be combined to create a “unique genome” for any specific digital tool. These “dimensions of genes” are:
What is being done? (goal): creation, decision
Who is doing it? (staffing): hierarchy, crowd
Why are they doing it? (incentive): money, love, and glory
How is it being done? (structure/process): collection, collaboration, group decision, individual decision
To explore these questions, the authors combined data from three public repositories Digital Participatory Platforms (containing 50 platforms); OECD Guidelines for Citizen Participation Processes (16 digital tools); and the Collective Intelligence through Digital Tools (62 tools). These combined repositories created a dataset of 116 unique digital tools that existed as of November of 2022. The dataset created has a row for each of the 116 tools and columns for each of the “genes” or features that are recorded for each tool. The genes correspond to various answers to the four questions above (what, who, why, when, and how). Then they perform a series of different types of cluster analyses to explore Research Questions 1 & 2.
The first analysis, corresponds to the first research question (What are the main functions that digital tools provide for e-participation?). This analysis then is about clustering genes based on their interaction, which in the analysis is the frequency of co-occurrences of genes.
For this analysis, three major clusters were identified, with the first cluster having significant strength. The first cluster primarily involves functions of problem and solution identification. The second cluster is primarily about collective decision making. The third cluster consists of only two genes: co-funding and technology regarding donation.
The authors describe the results to the first analysis like this:
This result indicates that digital tools are predominantly designed for crowdsourcing (e.g., information, opinions, and funding) to assist policymakers in making informed decisions.
The second analysis, corresponds to the second research questions (What are the prominent clusters of digital tools?). This analysis is about clustering similar tools based upon their attributes, their genes.
For the second analysis, the authors find 5 clusters of digital tools. The first cluster contains 56 of the 116 tools. So as with the first cluster of tool functions, the first cluster here is particularly strong. The first cluster is fundraising and problem and solution identification. Given the size and strength of cluster 1, the authors identified 5 sub-clusters within cluster 1. These 5 sub-clusters are:
Crowd-sourced mapping tools
Interactive and place-based survey tools for collecting citizens' voices
Facilitate idea creation and deliberations for consensus building or collective action
Analytical tools
Co-funding tools
In addition to this large Cluster 1, the authors also identified an additional 4 clusters of digital tools. Cluster 2 focuses on drafting and decision-making. Cluster 3 is a collection of specialized decision-making tools. Cluster 4 tools are provided by the public and nonprofit sectors, which facilitate a wide range of processes, from identifying problems to decision-making. And, finally, Cluster 5 includes various tools provided by small and medium-sized tech firms with dedicated support teams.
In analyzing these clusters together, the authors settle on 3 main clusters of digital participatory tools, provide types from each of these clusters, and the list example from the repositories. Their Table 7 below summarizes these findings.
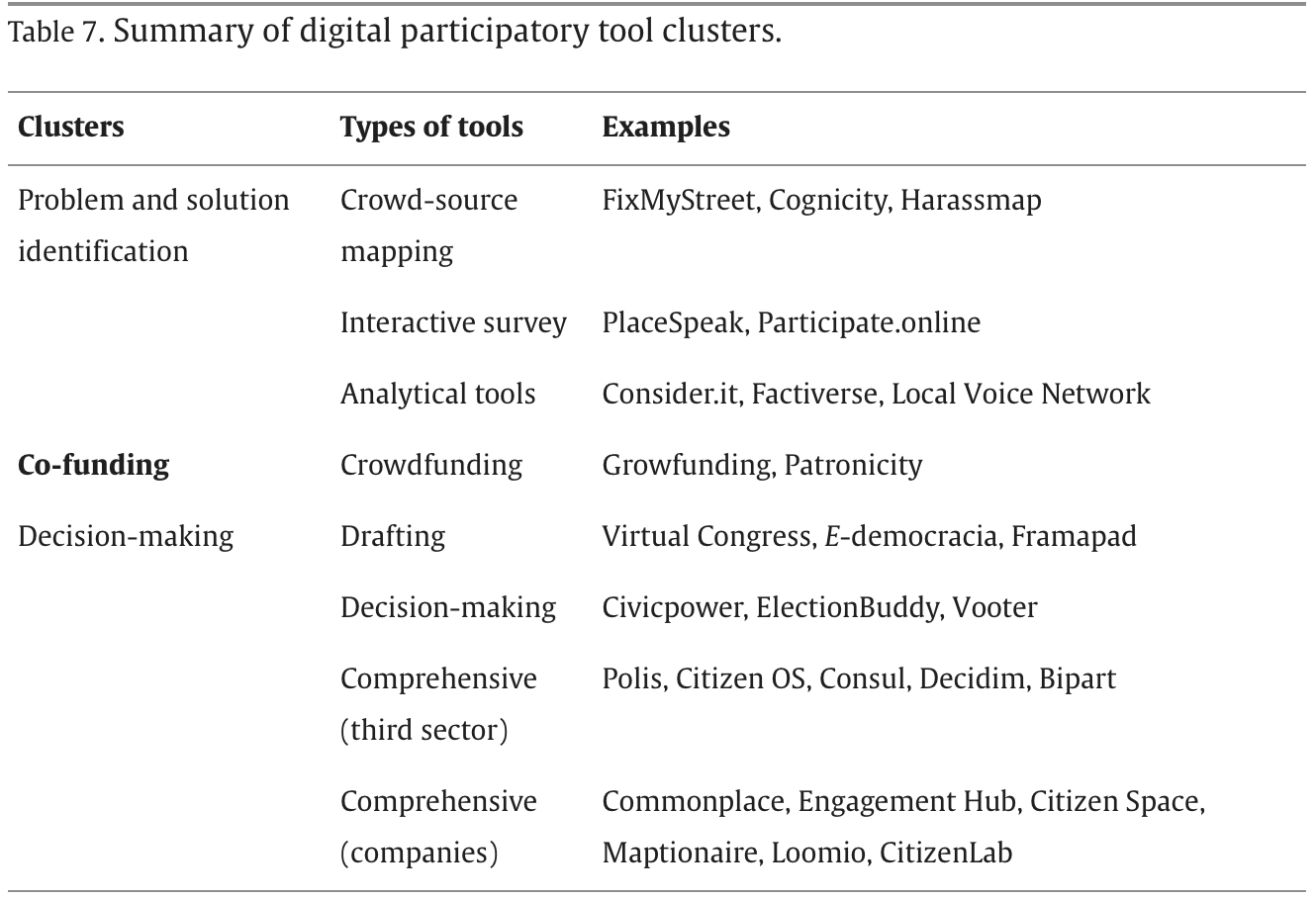
This table helps to capture the answers to the three main research questions the authors began with. The main prominent clusters of tools are: 1) problem and solution identification, 2) co-funding, and 3) decision-making. Within Cluster 1, main types of functions are: crowd-source mapping, interactive survey, and analytical tools. Cluster 2 contains crowdfunding as a key type of co-funding. Cluster 3 contains several functions as well including: drafting, decision-making, and comprehensive.
With the main clusters and functions identified, the authors also can examine research question 3: “Do digital tools potentially enhance inclusiveness, deliberation, and empowerment?” Here the authors argue that the tools they observe do seem to provide enhanced opportunities for inclusiveness and public deliberation, but the authors are more skeptical of empowerment. This finding comes directly from a few of the key takeaways offered by the authors:
“a relative absence of feedback loops, highlighting a gap in mutual interactions between citizens and government”
“only a few cases in our dataset included real-time monitoring and assessment systems”
“while citizens are encouraged to provide their data (e.g., comments, proposals, and votes) under e-participation, there is insufficient focus on how their voices influence decision making and policy action”
“However, given the ever-increasing availability of digital tools, our dataset does not cover new tools or prototypes that might contain advanced AI technologies… nor did we cover generative AIs with significant potential for human-machine collaboration.”
“Lastly, this article placed less emphasis on democratic aspects, such as equity, equality, freedom of expression, representation, civic education, and empowerment — all crucial for measuring the quality of e-participation.”
And in closing, the authors make a plea for more work in this direction
Therefore, we call for future research with a dedicated research design and theoretical framework that concentrates on exploring the democratic aspects of digital tools. This holds significance due to the pivotal challenge of digital inclusion in e-participation, a crucial aspect for fostering equality and empowerment.
“A Systematic Analysis of Digital Tools for Citizen Participation” was published in September of 2024 in leading academic journal Government Information Quarterly. On December 1, 2024, Goshi Aoki provided a response to some of these takeaways in the ArXiv preprint titled “Large Language Models in Politics and Democracy: A Comprehensive Survey.”
Large Language Models in Politics and Democracy: A Comprehensive Survey
Ask and you shall receive, 3 months later, the first paragraph of the abstract of “Large Language Models in Politics and Democracy: A Comprehensive Survey” :
The advancement of generative AI, particularly large language models (LLMs), has a significant impact on politics and democracy, offering potential across various domains, including policymaking, political communication, analysis, and governance. This paper surveys the recent and potential applications of LLMs in politics, examining both their promises and the associated challenges. This paper examines the ways in which LLMs are being employed in legislative processes, political communication, and political analysis.
As the title and the abstract above suggest, this essay provides a comprehensive review of how LLMs in particular are being used to influence politics and democracy. For our purposes, we are most interested in how LLMs influence democratic input, so not all the sections covered are equally relevant. But, when one table can do so much to give you the high-level findings of each of the sections, it seems like this table should be the starting point.
So:
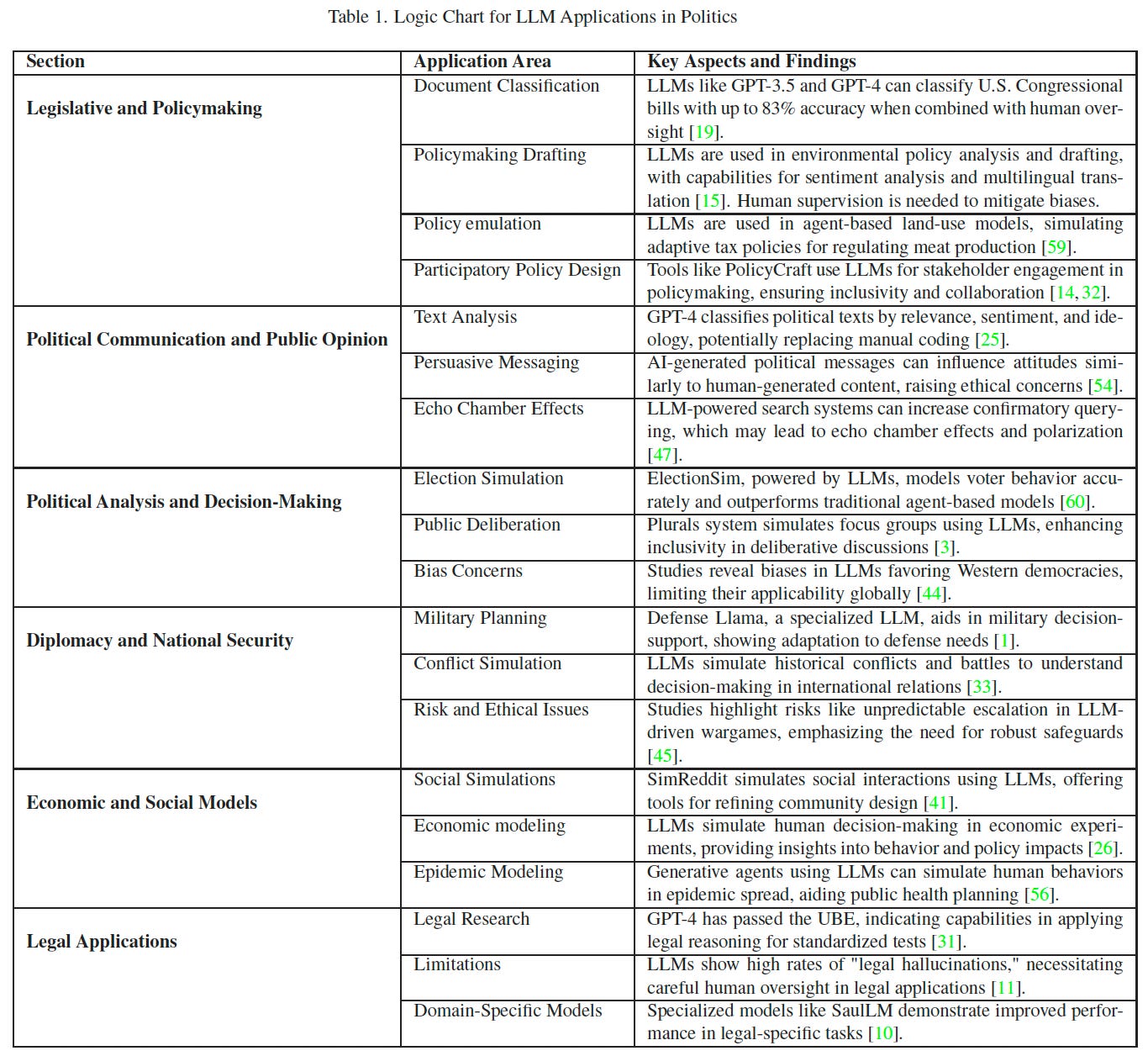
Before diving into the ways in which LLMs can be applied to politics and democracy as covered in this survey, I think it’s helpful to describe the “genetic makeup” of LLMs using the same sets of genes that the previous piece used to describe digital tools more generally. We run into a little difficulty as to what counts as a LLM, but I’ll briefly address this below.
The genetic makeup of LLMs looks something like the following:
Goals: co-production of knowledge; co-construction of policies and decisions
Providers: Private companies lead; Open Source plays a large role; some movement by Public sector
Rationales: problem identification, solution identification, drafting, decision-making, implementation, and assessment
Functionalities: Various human-human interaction, human-machine interactions, and Artificial Intelligence
What should make these particular genes noteworthy are the degree to which they capture the “general-purpose” nature of LLMs. That is LLMs have a wider set of capacities for use of digital tools than any of the digital tools explored in the previous study.
Now, we’re presented with one more challenge before we can turn to explore the impacts of LLMs on democratic input. And this one is pretty meta: what counts as democratic input? In particular, on what continuum of detailed and accurate must a simulation of the population be for it to count as a form of democratic input? When do simulations count as democratic input? For the purposes of understanding how LLMs might impact democratic input, I think simulations should be included. The idea is that a very accurate simulation of individuals or aggregated preferences would indeed be representative of the human preferences being simulated.
With all of this in mind, what is it that LLMs can do to influence democratic input?
This comprehensive survey lists 10 “application areas” which correspond somewhat loosely to the “function areas” from the digital tools paper. These application areas, along with a direct supporting quote from the survey itself, include:
Document Classification (“LLMs have shown promise in automating the analysis and classification of policy documents”)
Policymaking Drafting & Analysis (“real-time sentiment analysis and multilingual translation, which streamline decision-making in global governance contexts”)
Policy Emulation (“simulate institutional decision making” “LLM-powered agents could emulate realistic institutional behaviors such as incremental policy adjustments and stakeholder negotiation”)
Participatory Policy Design (“engaged stakeholders in iterative drafting and testing of LLM-generated policies”)
Text Analysis (“annotating political texts, classifying them by political relevance, negativity, sentiment, and ideology across multiple languages”)
Persuasive Messaging (“AI-generated messages can be as persuasive as human-generated content in influencing attitudes on political issues”)
Election Simulation (“LLMs can simulate and predict political dynamics”)
Public Deliberation (“Plurals, a system that leverages LLM-driven agents with personas to simulate focus groups.” “AI mediator that helped groups find common
ground on divisive topics. The AI-mediated statements were rated higher for clarity and fairness, reducing divisions and promoting consensus.”
Social Simulations (“GOVSIM platform simulates resource-sharing dilemmas, revealing that advanced LLMs, particularly when combined with communication and moral reasoning capabilities, can achieve partial sustainability in managing shared resources.”)
Economic Modeling (“LLMs as "Homo Silicus," computational analogs of humans for economic experiments. By replicating classic behavioral economics studies, the research showed that LLMs can exhibit context-sensitive behavior qualitatively similar to human data, offering a cost-effective and ethical alternative for piloting experiments and exploring economic theories.”)
Even just from these examples, we can see the ways in which LLMs are much more generally capable than any specific digital tool from the digital tool repositories explored in the previous study. One of the things about LLMs is that any one system can perform a wide array of functions, as demonstrated by the list of 10 applications above. However, as we shall we below, the impact and accuracy of LLMs in relation to democratic input is still limited. While LLMs show differential progress in capabilities across the application areas above, transformative cases like digital twins and voting reform still require improvements in LLM capabilities themselves.
With this in mind, let’s take a detailed look at two recent studies that empirically explore the ability of LLMs to simulate voting preferences at both the individual level and the societal level.
Two Empirical Cases: Digital Twins & Fair Voting
Large Language Models (LLMs) as Agents for Augmented Democracy
Gudiño, Grandi, and Hidalgo (2024) conducted a study exploring the use of LLMs as "digital twins" for an augmented democracy, where AI systems help represent citizens’ policy preferences. The researchers used data from Brazil’s 2022 presidential election, where 267 participants compared 67 policies from the platforms of candidates Lula da Silva and Jair Bolsonaro. Participants also provided demographic details, including political ideology, education, gender, and age. The study fine-tuned six LLMs, including GPT-3.5, LLaMA-2, and Falcon, using the Low-Ranking Adaptation (LoRA) method to train the models on this dataset, which is essentially a form of post-training fine tuning. The models were tested for their ability to predict individual preferences and aggregate population-level preferences, with results compared against a bundle rule—a heuristic assuming participants always favor proposals from the candidate aligned with their stated ideology.
Figure 1 from the study, visually displays this process:
The study found that fine-tuned LLMs consistently outperformed the bundle rule in predicting individual preferences, achieving accuracy rates of up to 76.7% with LLaMA-2 and 70.4% with GPT-3.5, compared to 71% for the bundle rule. When predicting preferences for pairs of proposals from the same candidate’s platform—a task where the bundle rule has no predictive power—the LLMs maintained accuracy levels between 65% and 77%. At the population level, probabilistic samples augmented with LLM predictions produced more accurate estimates of aggregate preferences than probabilistic samples alone.
This can be seen in Figure 2 from the study included below:
The study also highlighted important findings related to demographic factors influencing model accuracy. For example, the LLMs more accurately predicted the preferences of liberal participants compared to conservatives or centrists, and college-educated participants' preferences were better modeled than those of non-college-educated participants. Furthermore, while accuracy differences across gender were mixed (with some models favoring male participants and others favoring female participants), the models consistently performed slightly better for younger participants compared to older ones. These demographic variations suggest that while LLMs can capture nuanced preferences, their effectiveness may vary across different groups, which could present challenges for ensuring equitable representation in real-world implementations.
The findings suggest significant potential for using LLMs to enhance democratic participation by allowing citizens to express granular preferences beyond traditional party-line voting. By "unbundling" policy proposals from candidates’ platforms, LLMs can offer a more flexible approach to civic engagement, where individuals’ preferences on specific issues are represented in decision-making processes. However, the study also underscores the limitations of current LLM technology, particularly its struggles with nuanced or contradictory preferences and its dependence on the quality and diversity of training data. While the models showed promise in both individual and collective preference modeling, their performance varied based on participant demographics and specific tasks, such as predicting preferences across ideological divides or complex proposals.
Generative AI Voting: Fair Collective Choice is Resilient to LLM Biases and Inconsistencies
Majumdar, Elkind, and Pournaras (2024) conducted a study to evaluate how generative AI, particularly large language models (LLMs), can simulate voting behavior and support collective decision-making. The researchers employed a factorial design to simulate voting scenarios using over 50,000 AI-generated personas in 81 elections, encompassing cases like the 2012, 2016, and 2020 U.S. national elections and a participatory budgeting campaign in Aarau, Switzerland. They assessed three LLMs—GPT-3, GPT-3.5, and Llama2—alongside traditional voting aggregation methods, such as utilitarian greedy and equal shares, across various ballot formats ranging from simple binary choices to complex preference ranking. (Utilitarian greedy refers to a voting method that selects the most popular projects sequentially until the budget is exhausted, while equal shares aims to ensure proportional representation by distributing voting power equitably across all participants.) The study found that binary voting systems achieved higher consistency between human and AI choices (82.3%) compared to complex ballots (4.5%-27.2%), revealing the challenge of complex ballots for AI performance. By emulating human voters and their decision-making processes, the research explored the viability of LLMs in approximating both individual and collective preferences.
Figure 1 from the study is provided below. It graphically displays the research process and results.
A critical component of the study involved addressing the challenges posed by low voter turnout. The researchers simulated scenarios where abstaining voters were represented by AI personas, finding that AI could recover lost consistency—restoring the alignment of outcomes with what would have been achieved if more humans had participated—by up to 53.3% under low-turnout conditions. This recovery was especially significant in voting scenarios with fewer options but less effective in complex participatory budgeting contexts with many alternatives. The equal shares method was particularly robust, maintaining proportional representation and consistency even with as much as 50% of voters abstaining. In contrast, utilitarian greedy methods were less resilient in preserving equitable outcomes, especially as the proportion of AI representatives increased. These findings demonstrated the importance of aggregation methods in effectively incorporating AI into democratic participation.
However, while the findings suggest that AI interventions could enhance democratic resilience, they also reveal significant limitations. The study showed that political ideology, race, and levels of political engagement introduced notable inconsistencies. For example, white participants exhibited a 33% higher rate of inconsistency between their choices and AI-generated votes compared to non-white participants, suggesting demographic biases in how well AI modeled voter preferences. Additionally, individuals who frequently discussed politics displayed 63.2% higher inconsistency in AI alignment compared to those who did not, highlighting the difficulty of modeling nuanced or strongly held political beliefs. While conformity biases—such as preferences for environmental projects—positively influenced consistency, these unconscious biases tied to demographic and ideological factors demonstrated how LLMs can struggle with equitable representation.
Finally, while the researchers advocate for the adoption of fair voting methods like equal shares, they do not fully address the practical and ethical challenges of integrating AI into real-world voting systems. Issues such as data privacy, trust, transparency, and the governance of AI systems remain critical gaps in the discussion. The results underscore the potential of AI to support democratic innovations, but they also highlight the need for caution and comprehensive safeguards to ensure that these systems do not inadvertently undermine the democratic principles they are designed to support. As such, the study provides valuable insights but invites further exploration of the complexities and risks associated with AI-driven voting systems.
Taken together these two studies suggest promising innovative methods for improving democratic input through the use of LLMs for augmenting and simulating voter preferences. However, while LLMs perform well in some cases, complexity of the ballot, complexity of the aggregation method, and demographic and political biases all work against the current use of LLMs for voting. Despite these limitations, LLMs can already play an augmenting role for additional democratic input for information concerning individual preferences and simulating aggregate voting outcomes.
Federal AI Use Case Inventory
Finally, we have the US Federal AI Use Case Inventory. We’ve explored how the comprehensive survey characterizes the academic literature, but what uses is the government pursuing? A comprehensive assessment is beyond the scope of our goals for this review, but let’s take a brief looks at some this inventory and the scope of LLM use in service of democratic input.
In 2023, the US Government began tracking AI use cases that are deployed throughout the US executive branch.3 In the first year of the database, released at the end of 2023, over 700 AI use cases were reported. On December 15th of 2024, the Office of Management and Budget released the updated inventory of Federal AI Use Cases.4 The 2024 inventory lists over 1,700 cases and is available for download on GitHub.5
An initial evaluation of the dataset, supports the general picture that has been drawn in this literature review. That is, LLMs and generative AI tools are beginning to see adoption, testing, and iteration by governments. In this inventory of over 1,700 cases, at least 200 of the use cases explicitly note that they used LLMs or generative AI as part of their use case. The data on the use case are not always complete nor contain all the relevant details, so one can assume 200 is a lower bound here. This is a surprisingly large number to me given the overall newness of LLMs and generative AI tools.
Among the programs that utilized LLMs or generative AI, there are several uses that are directly about democratic input, and there are also quite a few that use simulations of human behavior as a source of input to decision making. Below are 10 representative examples that I pulled from the 2024 inventory:
One example is the Department of Homeland Security’s (DHS) Planning Assistant for Resilient Communities (PARC). The purpose of this program is creating “AI assistants in generating actionable, efficient plans to improve community preparedness.” The AI output for this program is a “Beta Release GenAI Plan Generator (based on OpenAI Models) produces hazards and mitigation plans.”
Another example is of the Department of Health and Human Services' (HHS) Risk Assessment Model (RAM) for the National Risk Index. The purpose of this program is “in tracking program progress and engaging stakeholders in risk assessments.” The AI output for this program is “reporting tools outputs for monitoring and visualization of risk data.”
The DHS has another project called AI/LLM to Generate Testable Synthetic Data. The purpose of this program is to create “test data to simulate real-world scenarios” and enhance “the ability to validate and test AI models efficiently.” Its AI outputs include generating “synthetic test data that mimics human behavior or scenarios.”
HHS has another project called Using Generative AI for Stance Analysis of Public Inputs. The purpose of this project is to simulate “public sentiment and stances of policy issues based on input analysis.” The AI outputs include “summary insights and stance classifications.”
HHS has another project called Reddit Post Analysis for Sexual Health Using LLMs. The purpose of this project is to simulate “patterns in user behavior to identify trends in sexual health discussions” and to provide “actionable insights for public health outreach.” The AI outputs of this project include “analyzed trends and topic classification based on Reddit discussions.”
DHS has another project called Human Interaction Simulation in Emergency Response. The purpose of this project is to model “human decision-making in emergencies for training and scenario analysis.” The AI outputs are “simulated decision trees and response pathways.”
HHS has another project called Behavioral Simulation for Public Health Messaging. The purpose of this projects is to simulate “human behavior to evaluate the impact of public health campaigns” and refine “messaging strategies to improve outreach and effectiveness.” The AI outputs are “behavioral response models and recommendations for messaging improvements.”
The Department of Housing and Urban Development (HUD) has a project called Community Engagement Modeling Tool. The purpose of this project is to simulate “community feedback to predict engagement levels in housing projects.” The AI outputs are “engagement metrics and recommendations for community outreach.”
The Department of the Treasury has a program called Virtual Environment for Policy Simulation. The purpose of this project is to simulate “economic and policy scenarios to assess potential impacts.” The AI outputs include “policy outcome predictions and economic impact assessments.”
The United States Agency for International Development (USAID) has a program called AI-Driven Social Behavior Modeling for Diplomacy. The purpose of this program is to simulate “social behavior to improve diplomatic strategies and international collaboration.” The AI outputs include “behavior models and insights for diplomatic decision-making.”
While none of these examples involve voting, we see several examples of LLM systems being deployed in various of the application areas identified in the comprehensive survey examined earlier. These applications, from the list of previously identified 10 “application areas” from the comprehensive survey include: policy emulation, participatory design, text analysis, public deliberation, social simulations, and economic modeling. In future work, it would be useful to assess the effectiveness of some of these application areas as evidence that complements the effectiveness of voting tools.
Conclusion
This living literature review has explored how technology has altered democratic input. We began with the example of voting. Voting is particularly instructive because while it has changed significantly overtime, the basic mechanics have remained roughly the same for at least 150 years in the US. Essentially, citizens are given the opportunity to vote for a representative at somewhat regular integrals. Tallies are taken from paper and digital ballots and a winner is declared.
However, voting is not the only method of democratic input. We explored a systematic account of the digital tools that enable additional forms of these inputs to democracy. These digital tools were categorized by their “genes” (goals, provider, and function). These tools provide new pathways to provide feedback to the government. However, one of the key findings was a relative absence of feedback loops that allow for a genuine process of integration of feedback by the government itself. This account also is clear in its lack of attention to advanced AI systems.
Following this, we explored a comprehensive survey of the ways in which advanced AI (in particular LLMs) is already being deployed towards politics and democratic input. This comprehensive survey identified at least 10 application areas for LLMs including: document classification, policymaking drafting and analysis, policy emulation, participatory policy design, text analysis, persuasive messaging, election simulation, public deliberation, social simulations, and economic modeling.
From here, we turned to two specific studies from the comprehensive survey that explore voting and policy preference elicitation in particular. Here we found attempts at using LLMs as digital twins at the individual level and election simulations at the societal level to assist in providing additional forms of democratic input. While both of these methods show promise in improving the quality and amount of democratic input, the low accuracy of digital twins capturing individual policy preferences and LLM biases in quality of prediction present major obstacles to the adoption of LLMs as voting mechanisms.
Finally, we explored the 2024 US Federal AI Use Case Inventory to examine what forms of democratic input and citizen participation were being created, trialed, and implemented by US Federal Agencies. Here we found over 1,700 use cases with at least 200 of them explicitly working with advanced AI systems such as LLMs. From within this set of 200 cases, we identified 10 specific use cases that focused on democratic input and citizen participation which included: planning assistants, risk assessment models, generate testable synthetic data, stance analysis, emergency simulation, behavioral simulation, and policy simulation.
Taken together, we see a clear co-evolutionary relationship between technological innovation and democratic input. In particular the internet and various digital tools have enabled a myriad of new forms of democratic input, however much use of these early digital tools has not been fully integrated into a continuous feedback process. LLMs in particular seem poised to apply general purpose tools to democratic input. While not quite ready to provide individual digital twins, the comprehensive survey identified at least 10 general application areas where LLMs are beginning to be used by governments as a source of democratic input. Additionally, all sorts of AI-tools are being created and trialed by the US Federal Government to make further use of AI to identify individual and collective preferences.
Finally, as AI capabilities continue to dramatically increase, one should expect that the ability to accurately ascertain individual policy preferences and accurately aggregate them will continue to improve. As these capabilities arise, they will present important questions as to whether democratic representation is best instantiated in the form of elected human representatives, or if improved forms of direct democracy can become more effective, efficient, and equitable at delivering the benefits from democratic forms of governance.
References
Aoki, G. (2024). Large Language Models in Politics and Democracy: A Comprehensive Survey (Version 1). arXiv. https://doi.org/10.48550/ARXIV.2412.04498
Gudiño-Rosero, J., Grandi, U., & Hidalgo, C. A. (2024). Large Language Models (LLMs) as Agents for Augmented Democracy. https://doi.org/10.48550/ARXIV.2405.03452
Majumdar, S., Elkind, E., & Pournaras, E. (2024). Generative AI Voting: Fair Collective Choice is Resilient to LLM Biases and Inconsistencies (No. arXiv:2406.11871). arXiv. https://doi.org/10.48550/arXiv.2406.11871
Shin, B., Floch, J., Rask, M., Bæck, P., Edgar, C., Berditchevskaia, A., Mesure, P., & Branlat, M. (2024). A systematic analysis of digital tools for citizen participation. Government Information Quarterly, 41(3), 101954. https://doi.org/10.1016/j.giq.2024.101954
http://homepage.cs.uiowa.edu/~jones/voting/pictures/#dre
https://www.csg.org/2023/11/08/election-technology-through-the-years/
https://ai.gov/ai-use-cases/
https://fedscoop.com/federal-government-discloses-more-than-1700-ai-use-cases/
https://github.com/ombegov/2024-Federal-AI-Use-Case-Inventory
Thanks for sharing this research. A couple concern I’d have about many of the ideas for “democratic” engagement through LLMs:
1. Successful democracy probably requires an informed, engaged public. Civic engagement is important not only because of what it provides to the government (input and info from citizens) but also because it encourages citizens to take ownership of their government (and hopefully become more informed during the process). If I know that an LLM will voice my opinion (accurately) on my behalf whenever I skip an election (or town meeting), why should I take the time to research the issues and go to the polls/meeting?
2. Vulnerability to (perceived) manipulation. Even authoritarian governments typically hold elections and engage in some performative displays of apparent democracy. The more complicated a system of democratic input is, the harder it is for the public to understand and monitor it. How can there be transparency/outside monitoring in tallying votes or compiling public comments generated by LLMs? Is the public really going to believe in their legitimacy? I’d be extremely skeptical if my mayor said that an important decision for the city had been shaped by “democratic input” provided by LLMs. Ordinary democratic processes are also subject to manipulation, of course, but the attack surface seems much broader when you add LLM agents.